“Who here had any democratic input into ChatGPT?” Catherine D’Ignazio asked the audience at the Radcliffe Institute’s Knafel Center. “Nobody’s raising their hand, that’s strange,” she joked, looking out at the predominantly female crowd gathered for a half-day conference on gender and artificial intelligence. Amid laughter, a lone voice piped up from the back of the room to call ChatGPT’s development “the greatest heist of human intellectual property in the history of the world.”
“We were not consulted,” agreed D’Ignazio, MIT associate professor of urban science and planning. “The current way that technology development is proceeding is extremely non-democratic.” And so, about 20 minutes into “Gender and AI: Promise and Perils” (with perils notably plural)—an event hosted by the Radcliffe Institute and co-sponsored by the Harvard Kennedy School (HKS) Women and Public Policy Program—the stakes of the gathering were made unambiguously clear.
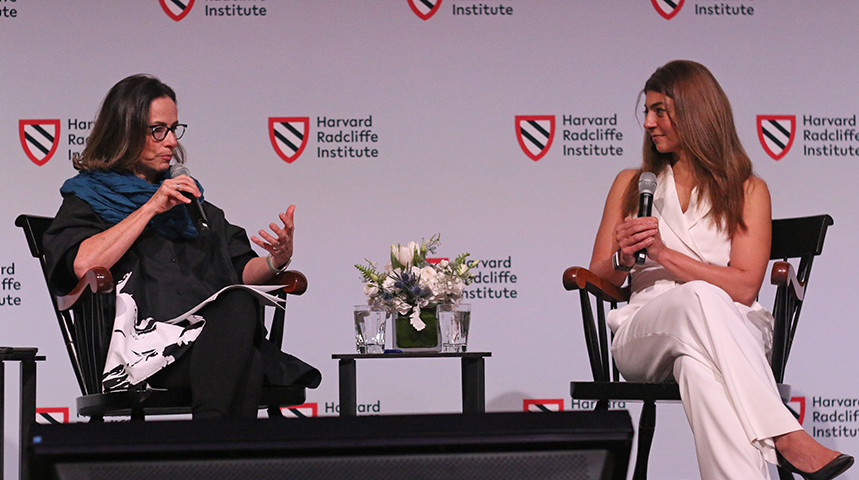
The April 11 conference explored how gender may affect “the creation, operation, and impact of cutting-edge technologies” and forayed into disciplines ranging from computer science to ethics and policy. Divided into three sessions, its format followed the stages of AI development: how algorithms are trained (“Inputs”); how the resulting systems can help solve problems facing women and marginalized communities (“Outputs”); and how AI should be governed (“Policy and Advocacy”).
“Garbage In, Garbage Out”
Smith professor of computer science Finale Doshi-Velez, moderating the first session, summarized the root cause of AI bias quite succinctly. “Garbage in, garbage out,” she said. “If the data have problems, the model will have problems.”
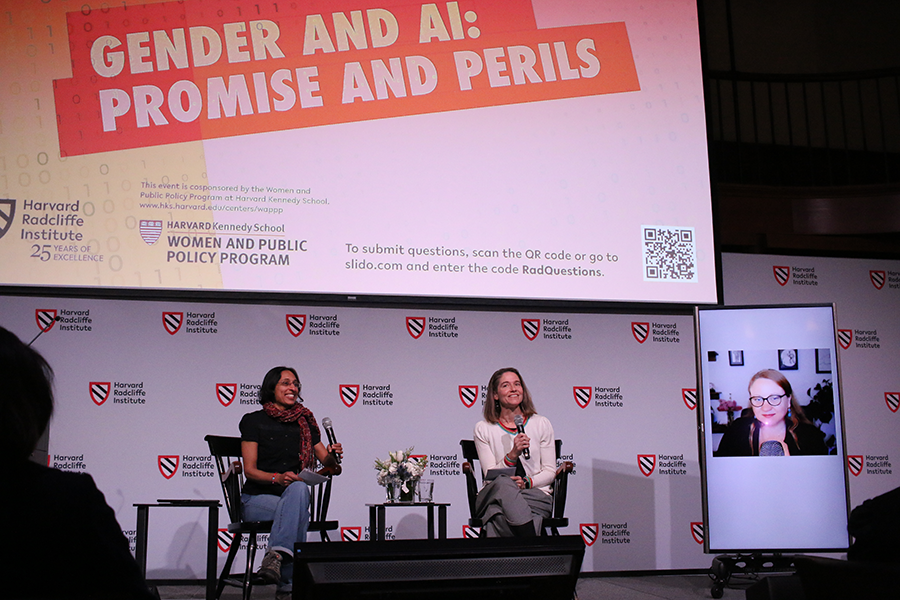
But algorithmic bias extends beyond data to what Doshi-Velez termed “structural inputs.” Access to resources powering AI (or “compute,” a term encompassing hardware such as computer chips and data storage centers) dictates what kinds of models are built and what problems they’re designed to solve. Today, a handful of large companies command the resources to train and deploy large-scale AI models. “These are the stakeholders,” D’Ignazio, speaking at the first session, emphasized. “So, of course, the systems are going to be built in a particular way.”
D’Ignazio’s co-panelist Margaret Mitchell—who appeared on stage by way of a giant free-standing video screen—stressed the importance of “consent, credit, and compensation” for data creators. This includes not only artists whose works were used to train AI image generators but also regular internet users whose data is often fed into large-language models like OpenAI’s ChatGPT. Mitchell, a chief ethics scientist at a company building open-source AI tools, cautioned that “it’s not always the case that having diverse systems is better.” There is a trade-off, she explained, between maximizing diversity and minimizing harms when it comes to providing AI models with training data. Companies might be eager to incorporate data from historically underrepresented groups, and willing to obtain that data without consent, in the name of diversity. But marginalized groups—particularly from the Global South—might be wary of surrendering their data to systems that could be used to oppress them (for example, facial-recognition software). In the context of a global race for AI dominance, one of the few reliable ways to ensure your data is not misused might be not to hand it over at all.
Asked to envision alternatives to corporate-owned AI models, the panelists discussed small-scale, decentralized technology tailor-made to solve specific problems in marginalized communities—such as a tool D’Ignazio built in collaboration with journalists to identify cases of gender-based violence in news articles. Both speakers acknowledged, however, that compute costs remain prohibitive, and as D’Ignazio put it, “things related to inequality are literally not profitable to solve.”
Deploying AI for Good
Tackling inequality is precisely what “Outputs,” the conference’s second session, focused on. Five participants gave brief presentations about AI tools they’ve developed to solve a problem that disproportionately impacts women and minorities—in sectors ranging from healthcare to law and banking.
For example, MIT School of Engineering distinguished professor for AI and health Regina Barzilay discussed MIRAI, a deep learning model she built that predicts a woman’s risk of developing breast cancer by analyzing mammogram results.
In the world of work, MIT visiting innovation scholar Frida Polli, M.B.A. ’12, described her fairness-optimized AI tool that reduces gender and racial bias in hiring. “Unconscious bias training doesn’t work,” she said, referring to the split-second and often subjective assessments humans make when selecting a candidate. “We know we can’t remove bias from the brain. However, we can attempt to remove bias from AI.” Polli built a company around this tool in 2012, and in 2021 helped pass the first algorithmic bias law in the nation, in New York City.
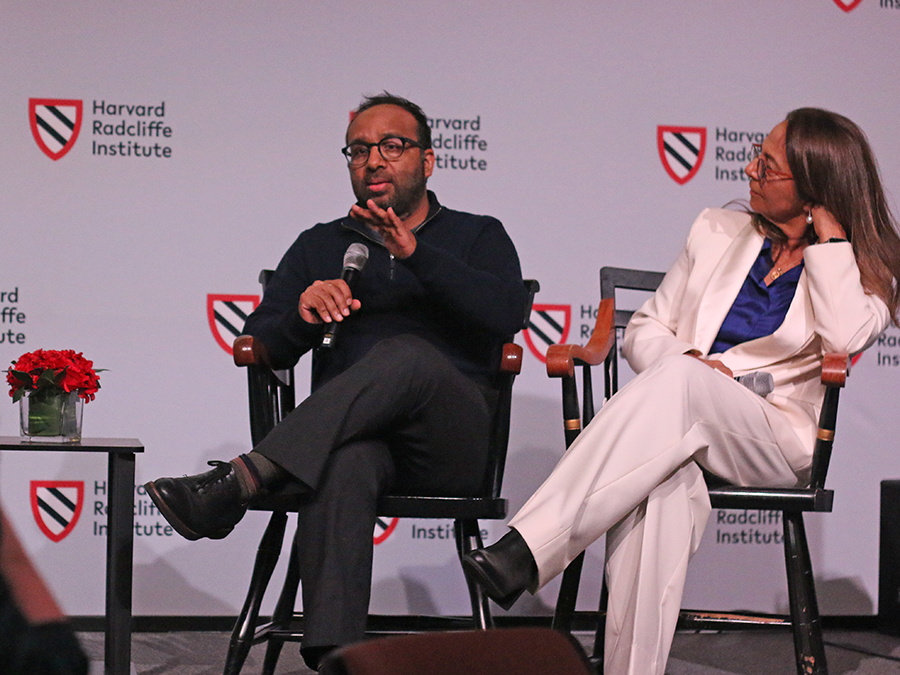
Keeping with the theme of human bias, HKS professor of public policy Sharad Goel zeroed in on the criminal justice system. In the U.S., prosecutors have to decide whether to bring charges against an individual who stands accused of a crime, and they often rely on police reports to make this determination. Goel’s AI-based tool “automatically mask[s] race-related signals”—such as names that may give away a person’s ethnicity, or a physical description that reveals the color of their skin—to help prosecutors make race-blind decisions.
AI Governance for the Trump Era
Researchers can design, test, and refine small-scale models to tackle particular problems, but what to do about massive systems already deployed at a national scale? This is where, at least in theory, government regulations come in. Three speakers at the “Policy and Advocacy” session tried to assuage fears about lackluster AI governance under the Trump administration by focusing on opportunities elsewhere.
The Berkman Klein Center’s Knight Responsible AI Fellow Rumman Chowdhury discussed her work with UNESCO on digital gender-based harassment, the European Commission’s open hearings on AI policy, and the UN’s plan to assemble “a scientific body to understand the impact of generative AI” as examples of international efforts to regulate the technology. Sandra Wachter, University of Oxford professor of technology and regulation, focused on European regulation, specifically the EU AI Act, which she called it “a good first step in acknowledging that AI very often will have a bias problem, and that [it’s] not something that’s arguable or up for debate.”
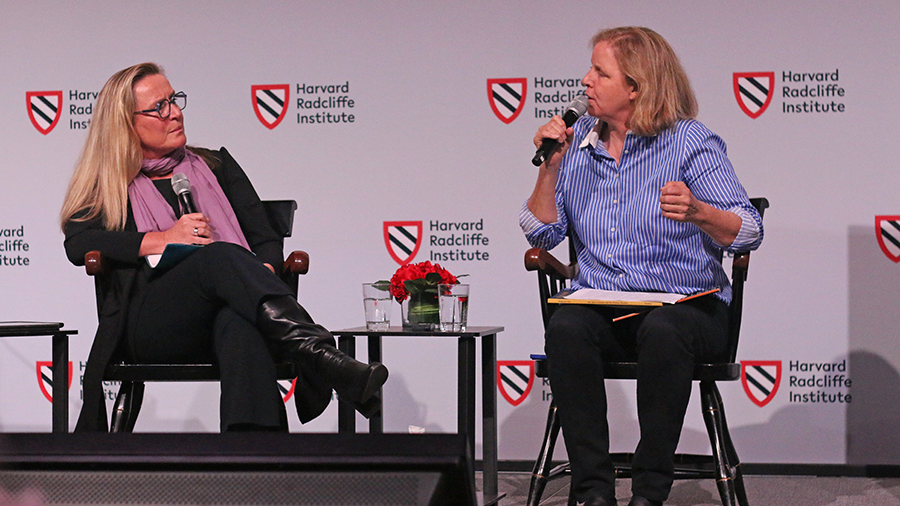
On the U.S. side, Walter Shorenstein Media and Democracy Fellow Megan Smith, the former chief technology officer and assistant to the president in the Obama administration, urged attendees to focus on “problems we already have” rather than worrying about frontier AI scenarios. She held up a copy of a 2017 report on the harms of algorithmic decision-making, which, she stressed, contained numerous mitigation strategies for AI-related challenges we’re now experiencing.
By the end of the conference, the collective mood turned a little somber. Participants had raised complex questions: Who is building today’s AI systems, and how are they addressing concerns about fairness, inclusive design, and environmental impacts? And if they aren’t, what might an alternative look like? But the resulting conversations did not yield clear answers.
In her closing keynote, Harvard Business School executive fellow Rana el Kaliouby offered one path forward. AI models have blind spots, many speakers reiterated, because the people designing them have blind spots. The best way to eliminate them is to gather as many diverse perspectives as early as possible in the model training process. El Kaliouby, who launched and later sold an emotion-detection startup, Affectiva, had a simple solution: put your money where your mouth is. “Who writes the checks determines who gets the checks,” she said. “And if we are investing in the same people and the same ideas over and over again, that’s going to be a problem.” Her new project, Blue Tulip Ventures, aims to widen the pool of ideas by financing early-stage AI startup founders—women in particular.
In bringing together a gymnasium full of women dedicated to making AI more inclusive, the organizers have taken a step toward El Kaliouby’s vision: to “humanize the technology before it dehumanizes us.”